Doing Big Data – Intelligently
14/12/2016
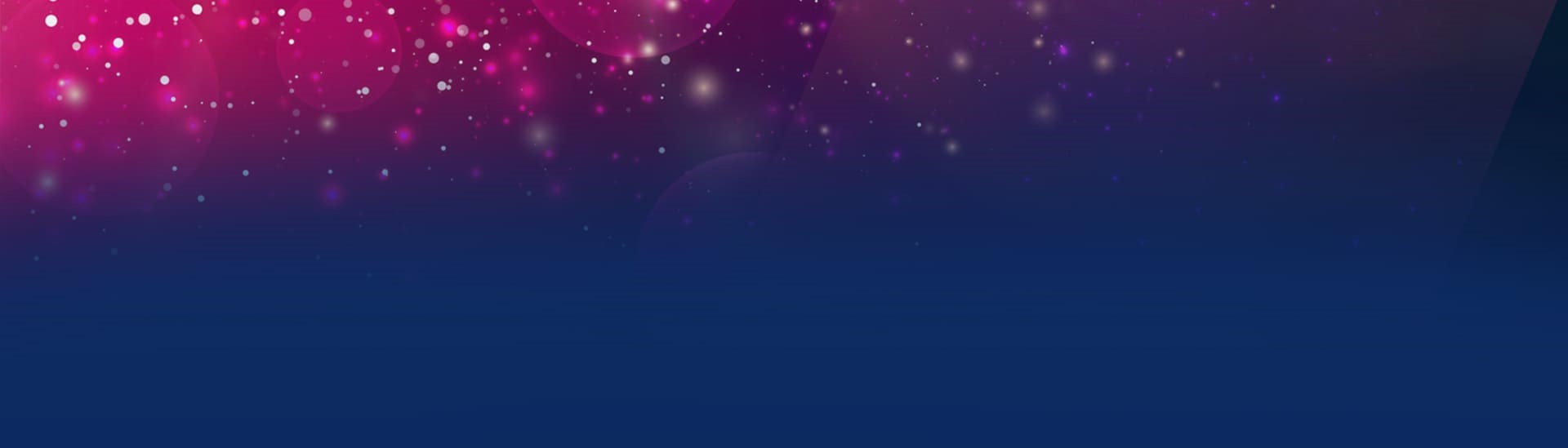
Last week we blogged about a term used by the Nobel Prize-winning author Daniel Kahneman in his book Thinking, Fast and Slow – WYSIATI (What you see is all there is). It refers to people wanting to see a complex world simplistically, whereas we would advocate that you have to understand (at some level at any rate) the complexity before simplifying – in other words “Simplicity on the far side of complexity”. Totally the opposite of WYSIATI – or “Simplistic on the near side of complexity”.
Many promises of Big Data fall into the area of simplistic on the near side of complexity. We hear Big Data is going to revolutionise our world from retail, financial services etc. through asset management and on into national security. This may be the case with unstructured data (i.e. free text), and possibly with structured data in some circumstances. It is obvious how a retailer might want to analyse all shoppers who purchased a certain washing powder for sensitive shin and run a promotion to also purchase sensitive skin cream and/or clothes made from materials for sensitive skin. Of course, retailers have been doing this for years – just not calling it Big Data.
However, there is a Big Question around Big Data revolutionising the Internet of Things or the Asset Management Industry. With sensor data coming from a myriad of equipment and machinery at the rate of every (typically) 100ms, we are being persuaded that it is necessary to capture every data point as if it has meaning – giving rise to vast so-called “data lakes” of petabytes and zeta bytes or whatever in size, with little hope of analysing it. It may revolutionise sales of Big Data solutions, but does it add value to understanding and analysis?
We came across a world-leading rubber moulding/sealing company in our travels over the past four years or so, and here we found this simplistic on the near side of complexity approach of “just put all the data into one place and run an algorithm to tell us what levers to pull” to get a perfect product. There was little interest in understanding, just “give me an answer”. They were generating large amounts of scrap – and still are.
More recently, we are working with a large transport organisation with many lifts in their buildings. We recognised that every data point did not hold value and that each data point was infected with electrical / mechanical noise that needed removal – in fact, through some intelligent analysis, we reduced the volume of rows by a factor of 400:1. Further analysis allowed us to enter the data into a proprietary time-series analysis platform and we were able to visualise normal behaviour hour by hour over several weeks, spot anomalies, see trends and patterns – all of which have never been seen before.
The Big Question – how would Big Data have provided such insight?
Categories & Tags:
Leave a comment on this post:
You might also like…
Keren Tuv: My Cranfield experience studying Renewable Energy
Hello, my name is Keren, I am from London, UK, and I am studying Renewable Energy MSc. My journey to discovering Cranfield University began when I first decided to return to academia to pursue ...
3D Metal Manufacturing in space: A look into the future
David Rico Sierra, Research Fellow in Additive Manufacturing, was recently involved in an exciting project to manufacture parts using 3D printers in space. Here he reflects on his time working with Airbus in Toulouse… ...
A Legacy of Courage: From India to Britain, Three Generations Find Their Home
My story begins with my grandfather, who plucked up the courage to travel aboard at the age of 22 and start a new life in the UK. I don’t think he would have thought that ...
Cranfield to JLR: mastering mechatronics for a dream career
My name is Jerin Tom, and in 2023 I graduated from Cranfield with an MSc in Automotive Mechatronics. Originally from India, I've always been fascinated by the world of automobiles. Why Cranfield and the ...
Bringing the vision of advanced air mobility closer to reality
Experts at Cranfield University led by Professor Antonios Tsourdos, Head of the Autonomous and Cyber-Physical Systems Centre, are part of the Air Mobility Ecosystem Consortium (AMEC), which aims to demonstrate the commercial and operational ...
Using grey literature in your research: A short guide
As you research and write your thesis, you might come across, or be looking for, ‘grey literature’. This is quite simply material that is either unpublished, or published but not in a commercial form. Types ...