Artificial Intelligence and Machine Learning Benefits? Is there any Evidence?
27/01/2020
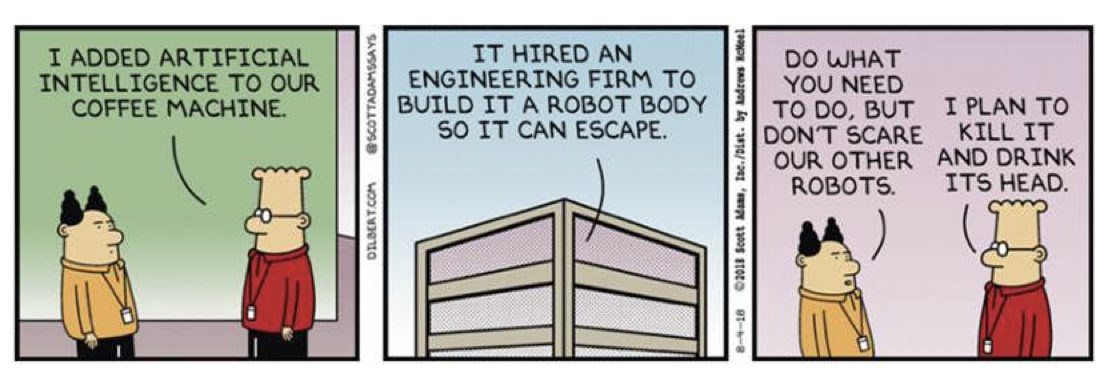
The world is awash with AI and ML programmes and projects – and marketing hype!
There is clearly a place for such applications – we heard recently about AI (actually it’s good old sophisticated image-recognition) beating experienced doctors in identifying breast cancer, we’ve heard about its use in some automated driving situations, etc.
However, although many organisations are developing and deploying these applications, it appears the world has yet to see any hard evidence that they are delivering benefits to stakeholders (apart from the developers and suppliers of such systems) either in terms of cost-savings/avoidance or increasing effectiveness / income.
There is overwhelming material out there about what they can deliver in the future – but not a lot on what has been delivered so far.
Our evidence for the lack of evidence comes from our own observations, as well as Dominic Cummings’ recent blogvert where he refers to a paper by Makridakis, Spiliotis and Assimakopoulos.
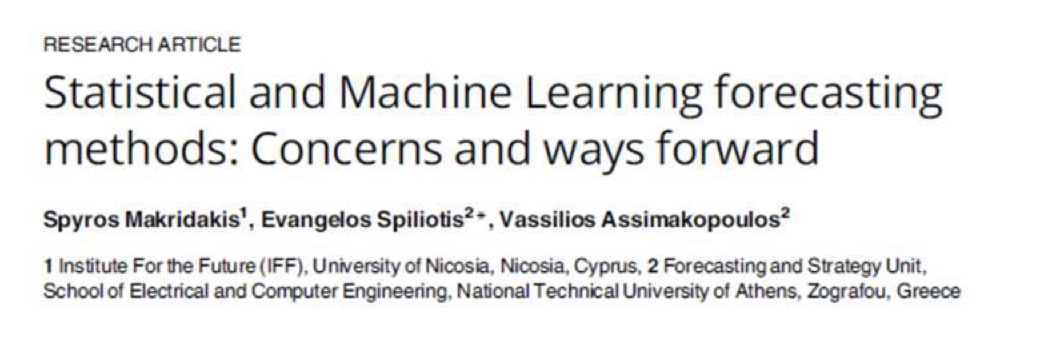
Some golden nuggets:
- “These studies contributed to establishing two major changes in the attitudes towards forecasting: First, it was established that methods or models, that best fitted available data, did not necessarily result in more accurate post sample predictions (a common belief until then)”
- “Second, the post-sample predictions of simple statistical methods were found to be at least as accurate as the sophisticated ones. This finding was furiously objected to by theoretical statisticians …”
- “A problem with the academic ML forecasting literature is that the majority of published studies provide forecasts and claim satisfactory accuracies without comparing them with simple statistical methods or even naive benchmarks. Doing so raises expectations that ML methods provide accurate predictions, but without any empirical proof that this is the case.”
· “In addition to empirical testing, research work is needed to help users understand how the forecasts of ML methods are generated (this is the same problem with all AI models whose output cannot be explained). Obtaining numbers from a black box is not acceptable to practitioners who need to know how forecasts arise and how they can be influenced or adjusted to arrive at workable predictions.”
- “A final, equally important concern is that in addition to point forecasts, ML methods must also be capable of specifying the uncertainty around them, or alternatively providing confidence intervals. At present, the issue of uncertainty has not been included in the research agenda of the ML field, leaving a huge vacuum that must be filled as estimating the uncertainty in future predictions is as important as the forecasts themselves.”
Work by Genevera Allen, Associate Professor at the Rice University in Houston, Texas also questions the lack of evidence – and, more importantly, the apparent lack of interest in even attempting to collect this evidence!
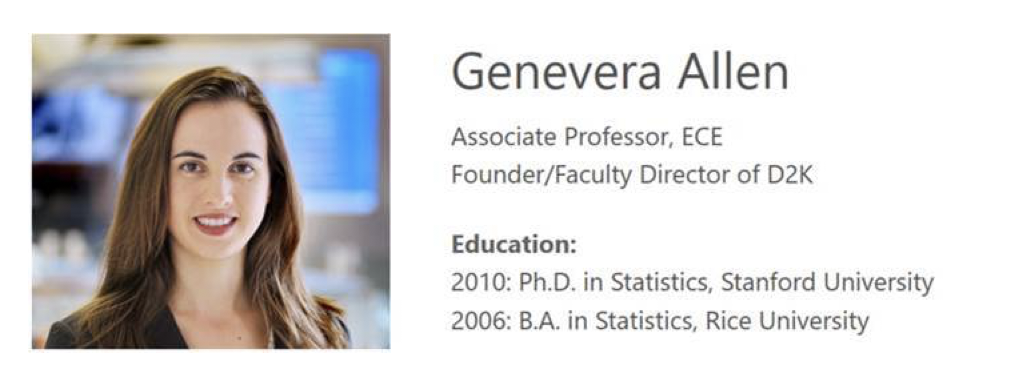
Some golden nuggets:
- ” … scientists must keep questioning the accuracy and reproducibility of scientific discoveries made by machine-learning techniques until researchers develop new computational systems that can critique themselves.”
- “The question is, ‘Can we really trust the discoveries that are currently being made using machine-learning techniques applied to large data sets?'” Allen said. “The answer in many situations is probably, ‘Not without checking,’ ….”
- “A lot of these techniques are designed to always make a prediction, they never come back with ‘I don’t know,’ or ‘I didn’t discover anything,’ because they aren’t made to.”
- “But there are cases where discoveries aren’t reproducible; the clusters discovered in one study are completely different than the clusters found in another,” she said. “Why? Because most machine-learning techniques today always say, ‘I found a group.’ Sometimes, it would be far more useful if they said, ‘I think some of these are really grouped together, but I’m uncertain about these others.'”
When we blogged about Matthew Syed’s “Black Box Thinking” in 2016, if you don’t have a properly constructed “closed loop” (in an engineering sense, and “open loop” in the Syed sense) learning system when experimenting with new applications, then we have no idea if we’ve actually improved things!
So, like Dilbert, you may want to be wary about where and how you apply AI and ML! And don’t start with the coffee machine!
Categories & Tags:
Leave a comment on this post:
You might also like…
Come to a virtual study session in May
What are virtual study sessions? These are online study sessions facilitated by Library staff, where you can study independently alongside other students via Teams. They are a great way for you to dedicate specific time ...
Getting started on your School of Management thesis
Writing a thesis, business plan, internship project or company project can be a daunting task, and you might have some uncertainty or questions around how to get started. This post will share some ideas and ...
Sustainability by royal request: Managing an event fit for a King
The Coronation of King Charles III on May 6th 2023, was watched by millions of people around the world with tens of thousands of people travelling to Central London to witness the pageantry firsthand. ...
Getting started on your Master’s thesis
Please note: This post is intended to provide advice to all students undertaking a thesis in the Schools of Aerospace, Transport and Manufacturing; Water, Energy and Environment, and Defence and Security. There is separate advice ...
Finding your tribe: “Joining the sustainability community was the best decision”
For students on Cranfield’s Sustainability Business Specialist Apprenticeship, community and camaraderie is a vital component for success. Designed in consultation with industry, the part-time Level 7 apprenticeship aims to deepen participants’ knowledge of the ...
“My sustainability studies gave me the confidence to take on Amazon”
Not everyone would have the confidence to challenge a big global power like Amazon but, for Colin Featherstone, Senior Technology Manager and Tech Sustainability Lead at Morrisons, his Cranfield studies equipped him with the ...