Performance Reporting Measures vs Performance Management Measures – Part 2
05/12/2019
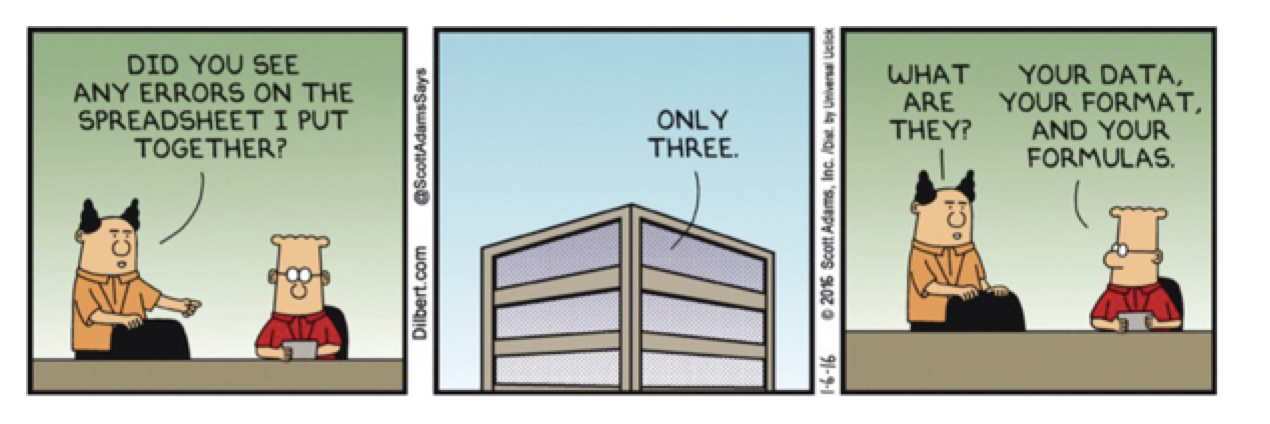
Another Problem with % Measures
You may have read my blog from last week comparing Performance Reporting Measures vs Performance Management Measures.
Performance reporting is littered with measures that may appear to carry meaning for some people, but in our observations, have been misleading and impenetrable to many. And certainly don’t help understanding nor how to improve!
Here are some examples of reporting measures that we introduced previously:
- % items completed: % implies a ratio – with a numerator and denominator. E.g. % Repairs Completed defined by (Number of Repairs Completed / Total Number of Repair Calls) * 100
- % completed within some timeframe: E.g. From a previous blog’s A&E Figures, we saw % A&E attendants seen in 4 hours or under.
- Complicated Measure Combinations: E.g. % Forecast Accuracy in Supply-chain
- Applying sophisticated statistical treatment to raw performance measures that only stats specialists can read: E.g. Exponentially weighted moving averages
- Statistical representation of a population of people or things: E.g. Electric Car Use by Country
There’s one more critical problem with % measures I didn’t mention last time. And this one is particularly mind-bending, even to some of those who have studied Maths!
You start to stumble across the problem when you start drilling down into sub-sets of the data to “better understand what is going on”. So, for example, regarding A&E data, you may want to drill down by hospital and by age-group. You do this at your peril!
But, to keep this light, we’ll select an alternative example from Wikipedia that you can all go take a look at – batting percentages over two years for two baseball players. We could have picked a cricketing example, but who knows what’s happening in the Test in New Zealand right now – well I said I wanted to keep this light!
So here are the baseball figures – the figures are (number of hits) / (number of “at bats”):

So looking at the individual year’s batting % in each of 1995 and 1996, you’d want to bet on David Justice. BUT! When you look at their combined % for the 2 years, you’d want to go with Derek Jeter. Confused?
I won’t explain this paradox here, since Wiki does a very good job of it – but it is well-known (to some mathematicians and stats guys) as Simpson’s Paradox. It happens because both the numerator and denominator can vary independently.
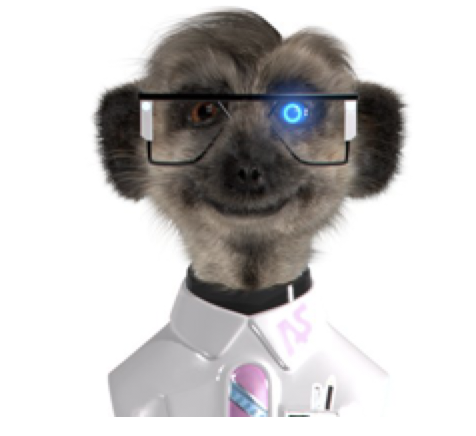
The ONLY way to resolve this is to have a clear PURPOSE for the business process (Wiki refers to STORY), which will guide the reader on whether to use the aggregated % or the component %s, OR to use an alternative measure altogether.
And I’m pretty sure Dilbert would encourage you to look at the underlying raw data – i.e. number of “at bats” and the “hits” separately (instead of, or, worst case, as well as %’s) if you really want to understand what’s happening!
Categories & Tags:
Leave a comment on this post:
You might also like…
Use academic libraries across the UK for free!
If you are studying or working away from our two University sites, you may find it more convenient to use a library service nearer to you. The SCONUL Access scheme gives all our staff and students free ...
A Journey in Manufacturing: From Egypt to Cranfield
Hi, my name is Karim Atteya, and I recently completed my MSc in Engineering and Management of Manufacturing Systems at Cranfield University. I’m originally from Egypt, and I chose Cranfield for ...
How do I reference… a foreign language item in the APA7 style?
Students sometimes ask if it is permissible to include non-English language items in their work and references. The answer is yes. You can include sources written in a language other than English provided that they ...
Inside the Thermal Power and Propulsion MSc with Dr Uyioghosa Igie
In our recent conversation with Dr. Uyioghosa Igie, Programme Director for the Thermal Power and Propulsion MSc at Cranfield University, we uncovered what makes this course such an exciting and valuable path for ...
Borrow fiction online – for free!
Everybody needs a break from work, and if you fancy reading or listening to some fiction or non-academic books, we have the app for you! Use the Libby app to borrow a host of online books ...
Researching IPOs in Bloomberg
Are you researching IPOs? Do you want to find IPOs on a specific index (eg S&P 500, or UK AIM Index) for specific dates? Then Bloomberg is where you should be looking. If you haven’t ...