Data documentation – what and why
22/09/2016
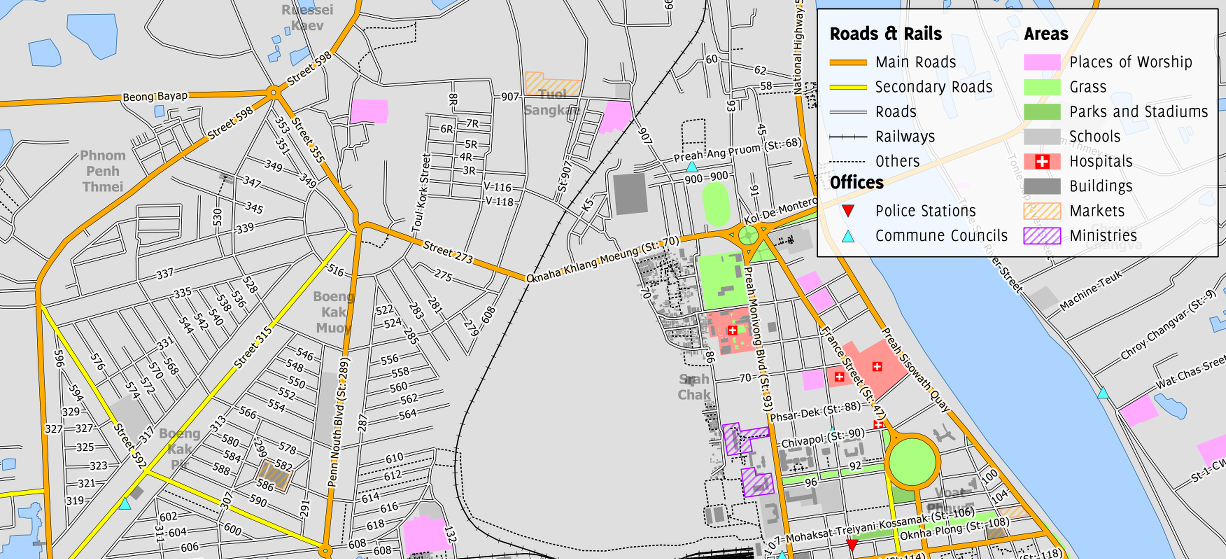
Just as a map becomes much easier to read when you have a legend explaining the symbols, data is much easier to comprehend when you have descriptive and contextual information. For example, while you’re using your research data, you’ll know what your variable names are short for, and what different missing value codes mean. But if you want to reuse it in a few years’ time, will you still be certain? Or if another researcher uses your data, will they be able to understand it without needing to ask you questions? Maybe you’ve experienced frustration yourself, when you want to incorporate existing data into your research, but it wasn’t made available with sufficient information and you’ve had to spend time contacting the creator.
Making documentation available alongside your data is therefore a key step to ensuring its long-term usability and value, both for you and others (which might lead to extra citations). Documentation can be as simple as one text file, in which you’ve noted all the key contextual information about the data and the project. Some elements you might want to include are:
- Study-level information
- Data collection methods, such as instruments used, sampling methods, scales, geographic/temporal coverage, etc.
- Data processing methods, such as validation, checking, cleaning, calibration procedures, etc.
- Data-level information
- Names, labels, descriptions, and units for all variables and fields.
- Explanation of codes, including reasons for missing values (not applicable/not provided/not recorded/error/etc).
With data-level information, you may be able to include enough information in the data files themselves (e.g. in table headings in a spreadsheet). Otherwise, you might want to store a separate explanatory file alongside your data file(s). You could use our template readme.txt file to note all the necessary elements you want to include, perhaps adding to it as you work through the project.
Or you might benefit from writing what’s called a “data dictionary”, basically a legend to your data. This great blog post on data dictionaries explains it in a nutshell, and highlights the benefits, whether sharing data with others (in or outside your project team) or just your future self. And remember that if you’ve entered this information into a tool such as SPSS, you should be able to export it to a file to store with your data (e.g. in SPSS v23, File > Display Data File Information > Working File > print, and print to pdf, or use the File > Export option in many screens to export to a more usable format such as txt).
So, when depositing your data in a repository, remember to include sufficient documentation for others (or your future self!) to understand it so no questions arise.
Image: City map by Mathieu Pellerin, CC-BY-NC-SA 2.0.
Categories & Tags:
Leave a comment on this post:
You might also like…
From Cranfield to CEO: an Emirati innovator’s lean journey
From the sands of Abu Dhabi to the lecture rooms of Cranfield University, one Emirati graduate has carved a remarkable path, blending academic rigour with entrepreneurial spirit. This is the story ...
Cranfield alumni hits 2 million views milestone with accessible archaeology YouTube channel
Cranfield alumni Rosie Crawford’s journey is nothing short of inspiring. While pursuing her undergraduate studies, Rosie created 'JustALittleRoo,' a free online access and outreach platform across YouTube, Instagram, and TikTok to share her experience as ...
From lean principles to lasting love: A Cranfield PhD journey
From the bustling streets of Turkey to Cranfield University, one graduate’s journey embodies the power of academic pursuit, industry connection, and unexpected life events. This is the story of Zehra Canan, ...
Finding economic data on Passport
One of the quickest and easiest ways to find global economic statistical data from a range of authoritative sources is to use Passport. Passport, a Euromonitor product, has both quarterly and annual historical data going ...
Level 7 apprenticeships support social mobility and address skills gaps
A recent article in The Times painted a misleading picture of Level 7 apprenticeships, accusing companies of sending senior executives on management courses, and diverting levy funding away from entry-level apprenticeships. The story fails ...
Want to improve your reading skills?
Are you starting to read through the mountains of journals, books or articles for your project or on your course reading list? Let’s start with a few myths about the reading process: You need to ...